In an earlier blog, I showed the video of my automated garden irrigation system that is powered by a couple of IoT devices with the control logic being handled by AWS IoT Analytics. In this post I’ll go a bit deeper into how it all works.
The overall system architecture looks like this – I have 2 micro-controllers powering the system, one handles all the environmental monitoring and one handles the flow of the water. I could do it all with a single micro-controller OK, this is just how my system happened to evolve. In both cases I’m using the ESP8266 and the Arduino IDE to write the code.
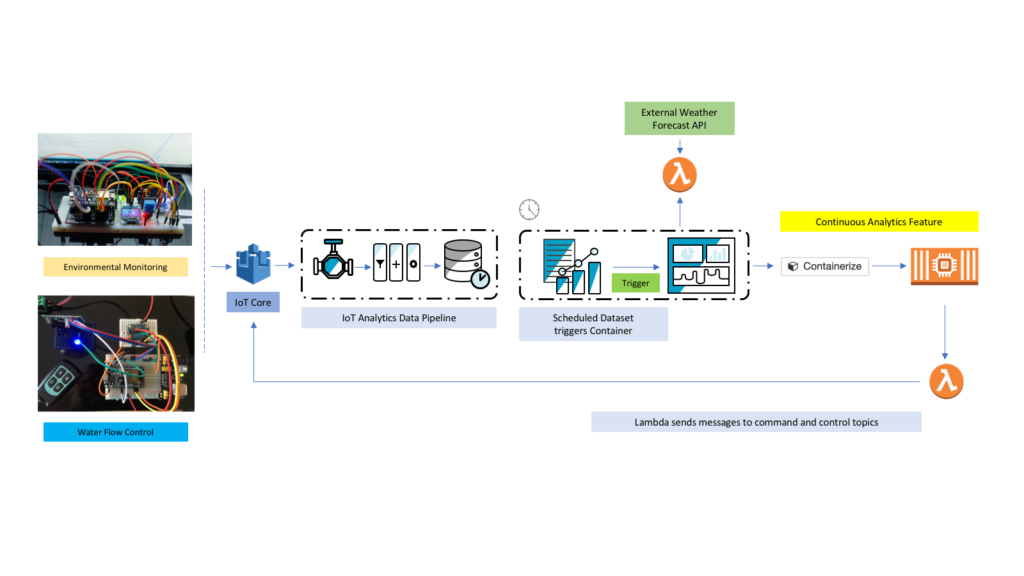
The problem can be broken down into getting the data, analyzing the data and then taking the appropriate actions depending on the analysis. In this project I’m going to use CloudWatch Rules to trigger Lambda Functions that control the water flow and use the Analysis to configure those Rules.
Data Sources
- Environmental sensor data on illumination & temperature
- Flow rate data from the irrigation pipe
- Future weather forecast data from darksky
Analysis
- Determine when to start watering by looking at the past illumination data
- Determine how much water by looking at the past temperature data
Command and Control
- Configure CloudWatch Rules to Trigger Water_On and Water_Off Lambda
- Send SNS notifications of planned activity
- Send SNS alarms if the water is flowing when it should not be
Before diving in to the analysis, a few words on where and how I’m storing all my device data.
My devices publish sensor data on a variety of MQTT topics, a sub-set of the topics I use would be;
rtjm/<DEVICEID>/weather/temperature
rtjm/<DEVICEID>/weather/humidity
rtjm/<DEVICEID>/photoresistor
rtjm/<DEVICEID>/infrared
rtjm/<DEVICEID>/barometer/pressure
rtjm/<DEVICEID>/barometer/temperature
The messages on all the topics are somewhat different, but typically contain a timestamp, a measurement and other small pieces of information.
I send all this data to a single channel and store it all in a single data store as I will use SQL queries in datasets and Jupyter Notebooks to extract the data I want.
The Analysis
The first step is to create data sets to collect all the data for the period we will analyze in the notebook. I’ve chosen to use 1 data set for the temperature data and another data set for the illumination data, but it would be equally possible to use a single data set with the right query.
What does my temperature data set look like?
SELECT * FROM telescope_data where __dt >= current_date - interval '7' day AND (unit='Celcius' OR unit='C') order by epoch desc
What does my illumination data set look like?
SELECT * FROM telescope_data where __dt >= current_date - interval '7' day AND unit='lumen' order by epoch desc
I’ve set both data sets to execute daily as the preparation step for the next stage of analysis.
The Notebook
The crux of this entire project is the Jupyter Notebook, so we’re going to look at that in some detail. The full code for the notebook is available here.
Let’s start with the basics, to read the contents of a dataset, we can use some code like this;
iota = boto3.client('iotanalytics')
dataset = "illumination"
dataset_url = iota.get_dataset_content(datasetName = dataset,versionId = "$LATEST")['entries'][0]['dataURI']
df_light = pd.read_csv(dataset_url,low_memory=False)
This reads the latest version of the dataset content (every time the dataset is executed, a new version will be generated) for the dataset called illumination and reads it into a panda dataframe called df_light.
df_light['datetime']=pd.DatetimeIndex(pd.to_datetime(df_light["received"]/1000, unit='s')) \
.tz_localize('UTC') \
.tz_convert('US/Pacific')
df_light.index = df_light['datetime']
This adds a datetime index to the dataframe using the ‘received’ column in the data and converts it to the appropriate timezone.
Next we do some analysis with this data to figure out when dawn is and when we should turn the water on. I’m not going to explain this in detail as really the analysis you will be doing is totally dependent on the actual problem you want to solve, but you can review the code for the notebook here.
Typically in my notebooks I plot the data I am working with so I can visually inspect whether the data aligns with my expectations. Here’s the illumination data plotted by the notebook for example;
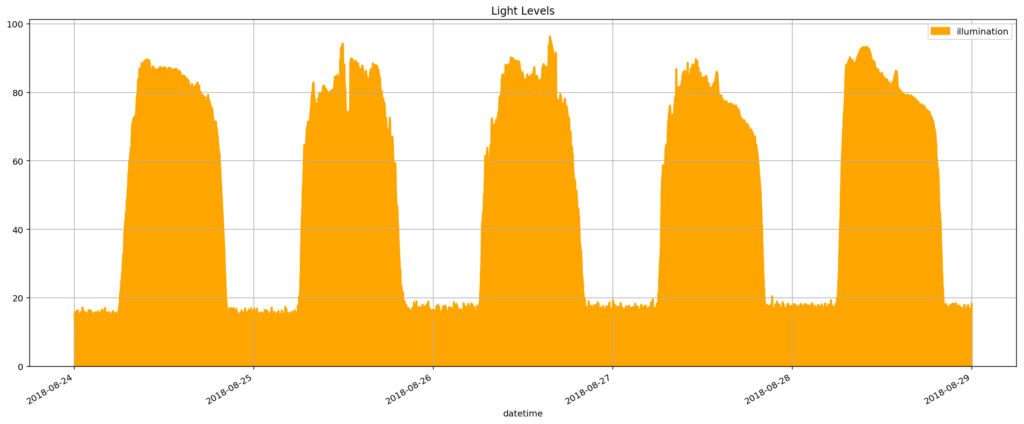
And here’s the temperature data from the other dataset.
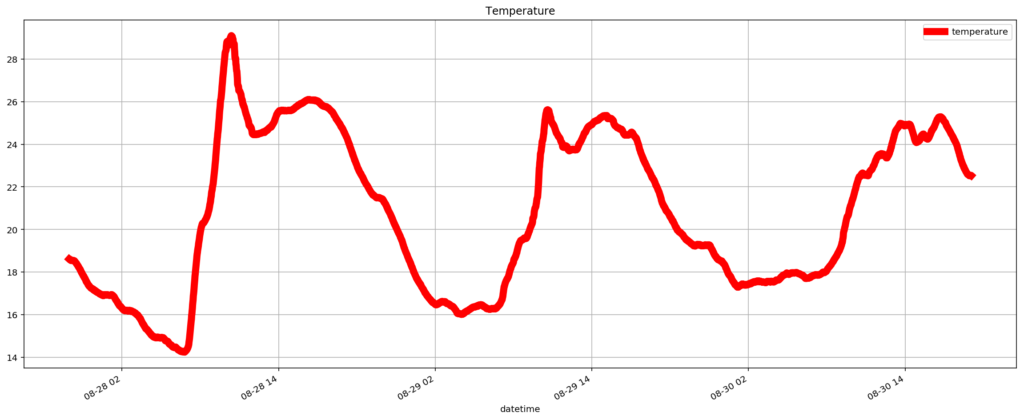
Looking at the notebook code, you’ll see that we distill this data down to a time to turn the water on and a time to turn the water off.
water on (local)= 2018-08-23 06:06:10
water off (local)= 2018-08-23 07:31:10
You will recall I mentioned we would look at the weather forecast to determine if it was going to rain or not? How does that work?
lambdaClient = boto3.client('lambda')
response = lambdaClient.invoke(FunctionName='IsItGoingToRain')
result = json.loads(response['Payload'].read().decode("utf-8"))
willItRain = result
I’ve encapsulated the ‘IsItGoingToRain’ into a Lambda function that is executed by the notebook and this beings me to an important but sometimes overlooked point – I can use the entire AWS SDK from within my notebook and this gives me a great deal of flexibility to design a solution that leverages many other services. This lambda function is really simple, the code looks like this;
import json
from urllib.request import urlopen
def lambda_handler(event, context):
url = "https://api.darksky.net/forecast/<REDACTED>/<LAT>,<LON>?units=si&exclude=currently,flags,alerts,minutely,daily"
response = urlopen(url)
weather = json.load(response)
hourly=weather["hourly"]["data"]
willItRain=False
for hour in hourly:
if ( hour["precipIntensity"] > 3 and hour["precipProbability"]>0.8) :
willItRain = True
return willItRain
Next the notebook leverages CloudWatch Event Rules to trigger another pair of lambda functions – one to turn the water on and one to turn the water off. Let’s take a look at the rule configuration to see how straight-forward that is as well.
ruleStatus = 'DISABLED' if (willItRain) else 'ENABLED'
cwe = boto3.client('events')
response = cwe.put_rule(
Name='water-on',\
ScheduleExpression='cron('+str(water_on.minute)+' '+str(water_on.hour)+' ? * * *)',\
State=ruleStatus,\
Description='Autogenerated rule to turn the water ON at the specified time')
response = cwe.put_rule(
Name='water-off',\
ScheduleExpression='cron('+str(water_off.minute)+' '+str(water_off.hour)+' ? * * *)',\
State=ruleStatus,\
Description='Autogenerated rule to turn the water OFF at the specified time')
The notebook goes on to publish the analysis back into another datastore, send messages to my phone etc, so please read the full notebook code here to get a sense of the variety of possibilities.
Great, so I have my notebook for analysis and I’ve tested it so that I’m happy, but how do I automate execution – it’s not very convenient having to manually run the notebook every time I want to adjust the irrigation and manual execution kind of misses the point of the project.
The key is to ‘containerize’ the notebook. This process is started by simply clicking on the containerize button you should see on the upper menu bar;
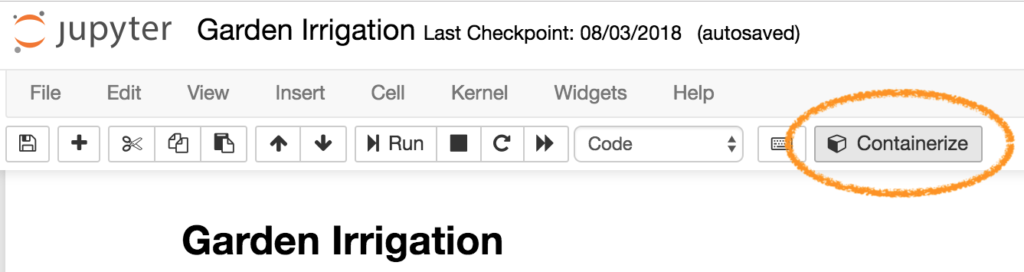
This process, launched at jupytercon 2018, allows you to package up your notebook into a docker image stored in an Amazon Elastic Container Registry repository and then you can use a container data set within IoT Analytics to execute the docker image on demand – either on a fixed schedule or triggered by the completion of another data set (which could be a SQL data set that prepares the data for the notebook).
The Result
Once a day my notebook is executed and determines when to turn the water on and off using both local environmental sensor readings and the weather forecast for the day ahead, the configuration drives CloudWatch Event Rules that invoke Lambda functions to turn the water on and off. The system has been up and running all summer without incident and the garden is still thriving.
UPDATE
Learn more about containerizing your notebook on the official AWS Iot Blog